The following Python script extracts the content of an article using Readibility (ported to Python), converts it to voice using the Amazon Polly service and finally sends the audio as a voice note to a given user in Telegram using Telethon (Telegram client for Python).
For running the script you will need to install the following Python packages:
pip install boto3
pip install awscli
pip install readability-lxml
pip install telethon
Also, you will need to create a AWS account. If you already have an AWS account, make sure that you have a user created in the IAM Management Console with the following permissions:
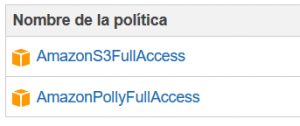
When creating this user, make sure you write down its ID access key
and its secret access key
. You will need them to configure the aws-cli
client.
With this Amazon credentials, you can configure the AWS client by executing the following command:
aws configure
In this step, you will need to fulfill the details with the user credentials you wrote down when creating it.
Now, you will need to create a Telegram API ID. For this, you can go to the Telegram section «Create an Application«. After following the steps described in the official documentation, you will obtain an API ID (it’s a number) and a API hash (it’s a string).
With these steps already completed, you can place all the needed details in the script and run it.
import boto3
import textwrap
import requests
import re
from readability import Document
from telethon import TelegramClient, events, sync
import time
import os
#--------------------------------------------------------------------
# Configuration
#--------------------------------------------------------------------
##### Article #####
# Define URL
url = "PLACE_THE_URL_HERE"
##### Telegram #####
# Telegram API credentials
api_id = PLACE_API_ID_HERE
api_hash = 'PLACE_API_HASH_HERE'
# Telegram user to send messages
telegram_user = "PLACE_TELEGRAM_USER_HERE"
# Create Telegram client
telegram_client = TelegramClient('session_name', api_id, api_hash)
telegram_client.start()
##### AWS configuration #####
# Get S3 session
session = boto3.Session(profile_name='default')
# Get polly client
polly = session.client('polly')
# Create a S3 client to retrieve the file content
s3 = session.client('s3')
# Define bucket name
bucket_name = 'PLACE_BUCKET_NAME_HERE'
#--------------------------------------------------------------------
# Get HTML from the article
#--------------------------------------------------------------------
# Get HTML
response = requests.get(url)
# Extract content with Readibility
doc = Document(response.text)
# Get article body
html_text = doc.summary()
# Regular expression to identify HTML tags, e.g.:
html_tag_re = r"<\\?[^>]+>"
# Remove HTML tags from the article body
text_only = re.sub( html_tag_re, "", html_text,)
# Send message to user pointing to the URL that is going to be converted
telegram_client.send_message(telegram_user, "Converting: %s" % url)
#--------------------------------------------------------------------
# Convert text to voice
#--------------------------------------------------------------------
# Start Polly task to save in a Bucket
resp = polly.start_speech_synthesis_task(OutputFormat='mp3',
OutputS3BucketName=bucket_name,
Text=text_only,
VoiceId='Enrique')
# Get Polly task
task = polly.get_speech_synthesis_task(TaskId=resp['SynthesisTask']['TaskId'])
# Monitor task status until it is completed
while task['SynthesisTask']['TaskStatus'] != 'completed':
# Wait 2 seconds between server poll
time.sleep(2)
# Get Polly task
task = polly.get_speech_synthesis_task(TaskId=task['SynthesisTask']['TaskId'])
# Print the status of the task
print("Task status: %s" % task['SynthesisTask']['TaskStatus'])
print("Task completed!")
#--------------------------------------------------------------------
# Retrieve file and send to Telegram user
#--------------------------------------------------------------------
# Regular expression to extract the key (file name) of the synthesized file
key_re = r'/([0-9A-Za-z-.]+)$'
# Search the regular expression in the OutputUri
regex_search = re.search(key_re, task['SynthesisTask']['OutputUri'])
# Take only the first group of the re (key)
file_key = regex_search.group(1)
# Get file name to store in local
title_sanitized = doc.short_title().replace('"', '')
title_sanitized = title_sanitized.replace(':', '')
file_name = "%s.mp3" % title_sanitized
# Download file from the bucket and store it in a MP3 local file
with open(file_name, 'wb') as data:
s3.download_fileobj(bucket_name, file_key, data)
# Delete remote bucket file
s3.delete_object(Bucket=bucket_name,Key=file_key)
# Delete local file
if os.path.isfile(file_key):
os.remove(file_key)
# Send MP3 file as a voice note to the telegram user
telegram_client.send_file(telegram_user, file_name, voice_note=True)
# Send signature
telegram_client.send_message(telegram_user, "Message sent from Romancero bot.")